Past projects
To provide an idea of the type of projects and activities Mooncake AI can help with, we list a few examples from the past.
Ethical reviewer
As part of the ethics board of a large energy infrastructure provider in the Netherlands, I assist in reviewing (AI and data) projects, to ensure that potential risks or challenges are identified and (1) either taken into account as the project is continued or (2) is discontinued entirely if the challenges can not be adequately addressed.
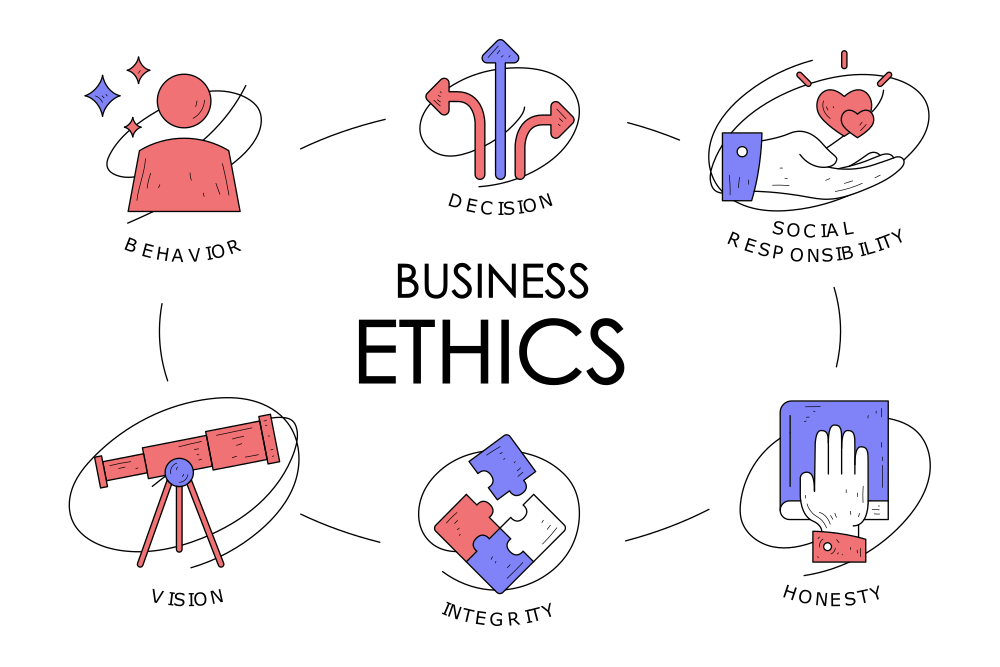
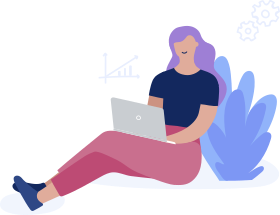
Development of Risk classification, risk assessment and risk monitoring
At a medium-sized financial organization, the AI governance process required for a solid risk classification (correctly recognizing AI-projects with medium to high risk potential). For this we developed and piloted an AI risk classification, focusing on risks associated with legal, as well as ethical and business risks. We then developed a follow-up assessment, which served to flesh out the exact details of the risk involved, and identifying potential measures to mitigate those risks. Part of the assessment, was to develop metrics to keep track of the risks, and pilots in which hypotheses about the effectiveness of the risk mitigation measures could be tested and validated. Ultimately, risk monitoring informs the effectiveness of the mitigation measures in real-time, alerting the organization as soon as a risk pops up unexpectedly.
Developing and Maturing a Responsible AI Capability @ Municipality
A medium-sized municipality in the Netherlands wanted advice and support in a path towards developing and maturing their AI capability in a responsible manner. The various viewpoints within the organization, as well as different levels of understanding of what AI is, what the opportunities and risks might be, and how to manage them appropriately, was bringing the discussions (and so also their development) to a halt. Through interviews and workshops, and getting to know the various viewpoints and departments, we developed a strategic roadmap to gradually build their capability, both in terms of technology and governance, by starting out small and simple, with low-hanging fruits. This will allow them to build trust in an evidence-based manner, and show how innovation can take place through controlled experimentation in a safe and secure environment.
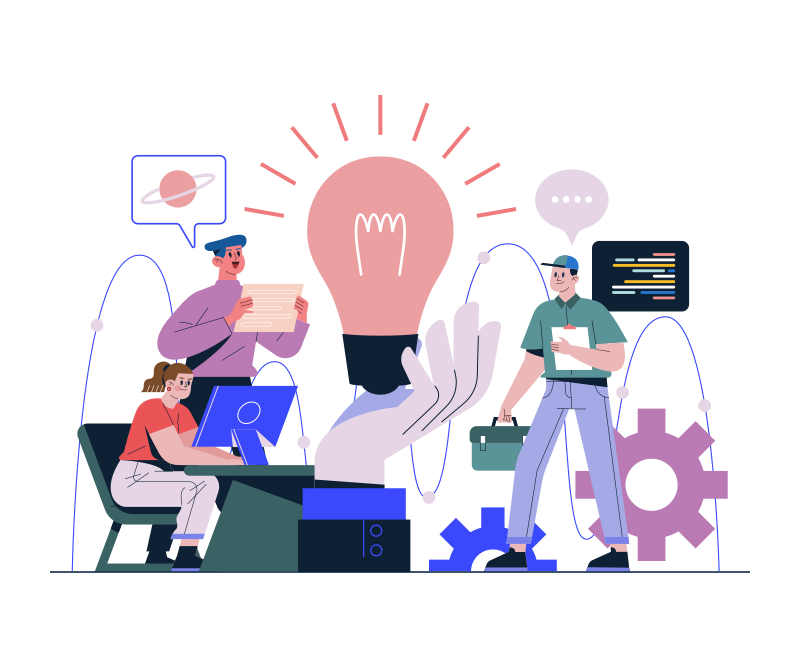
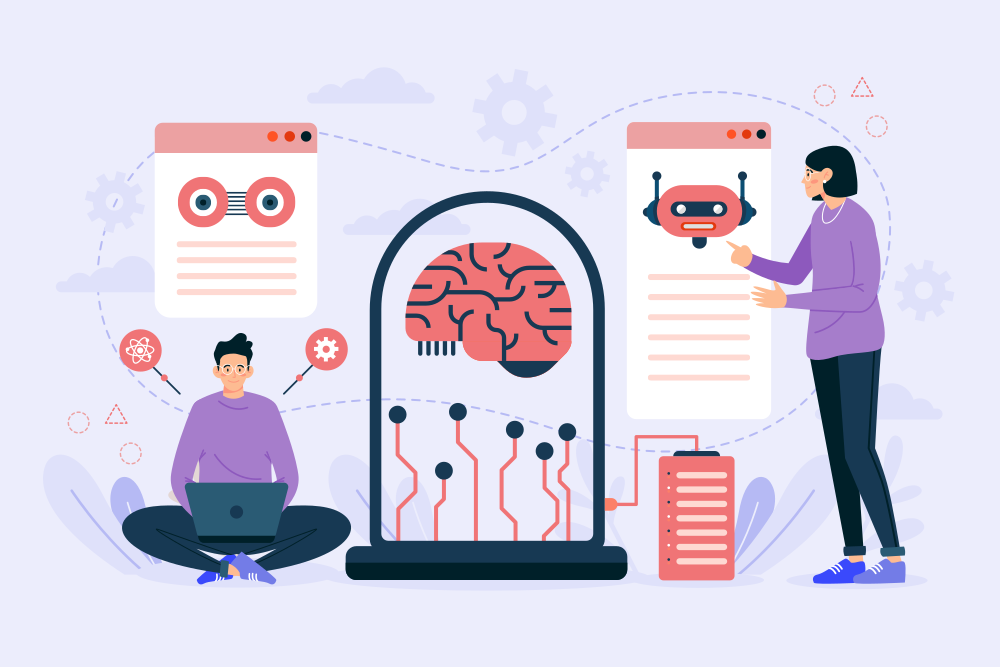
Responsible AI manifest and roadmap @ Financial Organization
My first project was at a medium-sized financial organization. Their Innovation Department had been working on an AI program for two years, and at times would run into ethical discussions. As they had no official policy, most of these discussions were resolved through individual and group decision making. To offer more consistency in these discussions, I assisted them through interviews, desk research and workshops, to draft up a set of principles and guidelines that would help them in the future. The advice I delivered, also included a roadmap towards a more integrated approach to Responsible AI across the organisation.
Selected publications
(complete list available on Google Scholar)
-
De Visser, E. J., Peeters, M. M., Jung, M. F., Kohn, S., Shaw, T. H., Pak, R., & Neerincx, M. A. (2020). Towards a theory of longitudinal trust calibration in human–robot teams. International journal of social robotics, 12(2), 459-478.
-
Peeters, M. M., van Diggelen, J., Van Den Bosch, K., Bronkhorst, A., Neerincx, M. A., Schraagen, J. M., & Raaijmakers, S. (2021). Hybrid collective intelligence in a human–AI society. AI & SOCIETY, 36(1), 217-238.
-
Peeters, M. M., Harbers, M., & Neerincx, M. A. (2016). Designing a personal music assistant that enhances the social, cognitive, and affective experiences of people with dementia. Computers in Human Behavior, 63, 727-737.
-
Van Diggelen, J., Neerincx, M., Peeters, M., & Schraagen, J. M. (2018). Developing effective and resilient human-agent teamwork using team design patterns. IEEE intelligent systems, 34(2), 15-24.
-
Mioch, Tina, Marieke MM Peeters, and Mark A. Neerincx. Improving adaptive human-robot cooperation through work agreements. 2018 27th IEEE International Symposium on Robot and Human Interactive Communication (RO-MAN). IEEE, 2018.
-
Vecht, B. V. D., Diggelen, J. V., Peeters, M., Barnhoorn, J., & Waa, J. V. D. (2018, June). SAIL: a social artificial intelligence layer for human-machine teaming. In International conference on practical applications of agents and multi-agent systems (pp. 262-274). Springer, Cham.
-
Peeters, M. M. M., van den Bosch, K., Meyer, J. J., & Neerincx, M. A. (2012). Situated cognitive engineering: the requirements and design of automatically directed scenario-based training. In Achi 2012, the fifth international conference on advances in computer-human interactions (pp. 266-272). XPS: Xpert Publishing Services.